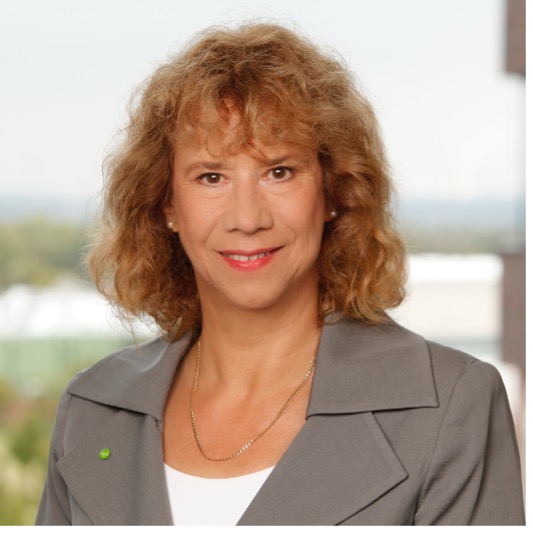
Katharina Morik
Lamarr-Institute for Machine Learning and AI TU Dortmund University
Embedding Machine Learning into Real-World Processes – A Time Ride through AI Applications
Abstract AI rapidly evolves due to the success of machine learning (ML) and hardware technology. Applications that were designed in the past can now be executed and more and more applications show up. Progress in the capabilities of ML models is published at a high rate, showing excellent performance on many benchmarks. Almost all processes in administration, customer communication, traffic, supply chain management, factory production, and science can be improved by ML. However, when it comes to introducing ML into real-world processes, we face – at least in Germany – a hesitating industry. This is partially due to a lack of trust in the technology, but there are additional factors that make deployment processes cumbersome. In this talk, some lessons from a variety of real-world applications will be presented ranging from natural language access to systems over an intensive care alarm system to applications in manufacturing. The lessons refer to the way in which we start a collaboration with industry and how we measure the impact of the deployment. The key to success is the knowledge about the complexity of ML and how to make good use of theoretical results without mentioning them. Since learnability relies on the representation of the training and testing data, feature construction and selection remain the decisive art of applications. The final step of actually putting the evaluated system to good use is up to the organization of the company. Here, companies will need to undergo some transformations if they want to reap the full benefits of AI.
Katharina Morik received her doctorate from the University of Hamburg in 1981 and her habilitation from the TU Berlin in 1988. In 1991, she established the chair of Artificial Intelligence at the TU Dortmund. She retired in 2023. She is a pioneer in bringing machine learning and computing architectures together so that machine learning models may be executed or even trained on resource-restricted devices. In 2011, she acquired the Collaborative Research Center CRC 876 “Providing Information by Resource-Constrained Data Analysis”, consisting of 12 projects and a graduate school. After the longest possible funding period of 12 years, the CRC ended with the publication of 3 books on Resource-Constrained Machine Learning (De Gruyter). She is a co-founder of the Lamarr Institute for Machine Learning and Artificial Intelligence, which is one of the six German National Centres of Excellence for AI Research. She has participated in numerous European research projects and has been the coordinator of one of them. She was a founding member and Program Chair of the conference series IEEE International Conference on Data Mining (ICDM) and has been a member of the steering committee of ECML PKDD for years. Prof. Morik is a member of the Academy of Technical Sciences and the North Rhine-Westphalian Academy of Sciences and Arts. She has been awarded Fellow of the German Society of Computer Science GI e.V. in 2019.
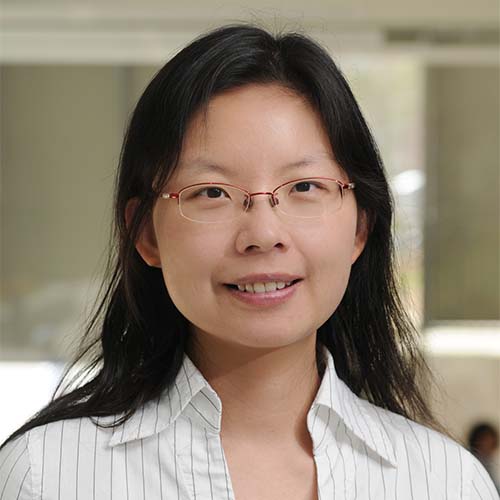
Jessica Lin
George Mason University
From Repetition to Insight: Shaping a More Interpretable Future through Time Series Motif Discovery
Abstract The rapid and continuous generation of massive amounts of data presents both significant challenges and exciting opportunities in how we manage, analyze, and extract valuable insights. Among the most complex and informative types of data are time series, which have become a central focus of research and application over the past two decades. In this presentation, I will explore unsupervised pattern discovery in time series data, with a particular emphasis on time series motif discovery. Time series motif discovery involves identifying recurring, similar patterns within a dataset over time. These motifs are essential for summarizing repetitive behaviors, uncovering hidden structures, and revealing meaningful semantics within complex time series data. Beyond their role in providing insight into the data, motifs serve as powerful building blocks for a variety of downstream tasks, such as classification, and anomaly detection, and enable us to build more effective, transparent, and interpretable models. In this talk, I will address the foundational challenges that arise in motif discovery. I will also highlight recent advancements that have made significant strides in overcoming these challenges. Finally, I will discuss the open problems, emerging trends, and how the latest technologies and methodologies are poised to shape the future of time series data mining and machine learning.
Dr. Jessica Lin is an Associate Professor in the Department of Computer Science at George Mason University. She received her PhD in Computer Science from the University of California, Riverside, in 2005. Dr. Lin’s research interests focus on the mining of massive time series and spatiotemporal databases. More specifically, she has published work on anomaly detection, frequent pattern (motif) discovery, contrast set, and rule mining, clustering, and visualization on time series and spatiotemporal data. She has worked on several collaborative projects in diverse disciplines, including medicine, activity recognition, national security, CPU manufacturing, finance, geoinformatics, and meteorology, with support from the National Science Foundation (NSF), Missile Defense Agency, Sandia National Laboratories/Department of Energy (DOE), U.S. Army, Naval Research Lab (NRL), and Intel Corporation. Dr. Lin has served as an Area Chair or PC member in top-tier data mining conferences, including KDD, PKDD/ECML, SDM, ICDM, and CIKM. She is an Associate Editors for the Knowledge and Information Systems Journal (KAIS), ACM Transactions on Intelligent Systems and Technology (ACM TIST), Pattern Recognition Journal, and the Big Data Research Journal.